Remote sensing techniques for land cover classification offer powerful tools to map and monitor Earth’s surface. You’ll find a range of methods, from optical satellite imagery to radar-based approaches. These techniques harness various parts of the electromagnetic spectrum, allowing you to analyze vegetation, water bodies, and urban areas. LiDAR technology provides detailed 3D maps, while hyperspectral sensors offer precise material identification. Machine learning and object-based analysis enhance classification accuracy, especially for complex landscapes. Multitemporal approaches track changes over time, and data fusion combines multiple sources for richer insights. Exploring these techniques will reveal a world of possibilities for understanding our planet’s ever-changing landscape.
Optical Satellite Imagery
Optical satellite imagery harnesses the power of visible and near-infrared light to capture detailed views of Earth’s surface from space.
You’ll find this technique invaluable for observing large areas quickly and efficiently. Satellites equipped with multispectral sensors collect data across various wavelengths, allowing you to analyze different aspects of land cover.
When you’re working with optical imagery, you’ll typically use bands in the visible spectrum (red, green, and blue) along with near-infrared.
These bands help you distinguish between vegetation, water bodies, and urban areas. You can create false-color composites by combining different bands, enhancing features that aren’t visible to the naked eye.
The resolution of optical imagery varies, from low-resolution images covering vast areas to high-resolution shots detailing individual buildings.
You’ll need to weigh the pros and cons between spatial, spectral, and temporal resolution when selecting imagery for your project.
Cloud cover can be a significant limitation, as optical sensors can’t penetrate clouds.
To overcome this, you’ll often use multiple images from different dates or integrate radar data. Despite these challenges, optical satellite imagery remains a cornerstone of remote sensing for land cover classification.
Hyperspectral Remote Sensing
While optical satellite imagery offers a broad view, hyperspectral remote sensing takes spectral analysis to the next level.
You’ll find that hyperspectral sensors collect data across hundreds of narrow, contiguous spectral bands. This detailed spectral information allows you to identify and differentiate between materials with greater precision than traditional multispectral imagery.
When you’re working with hyperspectral data, you’re fundamentally analyzing the unique spectral signatures of different objects.
These signatures act like fingerprints, enabling you to distinguish between various types of vegetation, minerals, and even man-made materials. You can use this data to detect subtle changes in ecosystem health, identify specific crop types, or locate mineral deposits.
However, you’ll need to be aware of the challenges that come with hyperspectral remote sensing.
The massive amount of data collected requires significant processing power and storage capacity. You’ll also need specialized software and expertise to interpret the complex spectral information effectively.
Despite these challenges, hyperspectral remote sensing continues to advance, offering you unprecedented insights into the Earth’s surface composition and condition.
LiDAR Technology
LiDAR technology revolutionizes remote sensing by using laser pulses to measure distances and create detailed 3D maps of the Earth’s surface.
It’s a powerful tool that can penetrate vegetation canopies and provide accurate information about terrain, buildings, and other structures.
When you’re using LiDAR, you’ll find it’s especially useful for applications like forestry management, urban planning, and flood risk assessment.
The technology works by emitting rapid laser pulses and measuring the time it takes for the light to return after hitting an object.
This allows for precise measurements of elevation and surface characteristics.
You’ll often see LiDAR data presented as point clouds, which are collections of millions of individual measurements.
These can be processed to create digital elevation models (DEMs) and other valuable products.
LiDAR’s ability to capture fine details makes it ideal for mapping complex environments like cities or dense forests.
One of LiDAR’s key advantages is its ability to operate day or night and in various weather conditions.
It’s also becoming more accessible as costs decrease and technology improves, making it an increasingly popular choice for remote sensing projects.
Radar-based Classification Methods
Radar-based classification methods offer a powerful approach to remote sensing, complementing other techniques like LiDAR.
These methods use active sensors that emit microwave signals and analyze the returned echoes to classify land cover types. You’ll find that radar systems can penetrate clouds and operate in various weather conditions, making them particularly useful for continuous monitoring.
When you’re working with radar data, you’ll typically use two main classification approaches: pixel-based and object-based.
Pixel-based methods classify each individual pixel based on its spectral properties, while object-based methods group similar pixels into segments before classification.
You’ll often employ machine learning algorithms, such as support vector machines or random forests, to improve classification accuracy.
One of the key advantages you’ll notice with radar-based methods is their ability to detect structural differences in land cover.
This makes them excellent for distinguishing between forest types, crop varieties, and urban structures.
You’ll also find that radar data can provide valuable information about soil moisture and surface roughness, enhancing your ability to classify and monitor agricultural areas and wetlands.
Machine Learning Algorithms
Machine learning algorithms have revolutionized remote sensing classification techniques, including radar-based methods.
You’ll find that these algorithms can process vast amounts of data quickly and accurately, making them ideal for land cover classification tasks. Common machine learning approaches include support vector machines (SVM), random forests, and neural networks.
When you’re working with SVMs, you’re using algorithms that find vital boundaries between different land cover classes in high-dimensional feature spaces.
Random forests, on the other hand, utilize multiple decision trees to classify land cover types, offering robustness against overfitting.
Neural networks, especially deep learning models, have gained popularity due to their ability to learn complex patterns from raw data.
You’ll need to weigh the pros and cons between different algorithms.
While neural networks can achieve high accuracy, they often require large training datasets and significant computational resources.
SVMs and random forests might be more suitable for smaller datasets or when transparency is vital.
As you implement these algorithms, you’ll also need to address challenges like feature selection, model tuning, and handling imbalanced datasets to achieve peak performance in your remote sensing applications.
Object-based Image Analysis
Object-based image analysis (OBIA) represents a significant shift from traditional pixel-based methods in remote sensing.
Instead of focusing on individual pixels, OBIA groups similar pixels into meaningful objects, allowing you to analyze images based on shape, texture, and contextual information. This approach mirrors how humans interpret visual information, making it more intuitive and often more accurate for land cover classification.
When you’re using OBIA, you’ll start by segmenting the image into homogeneous objects.
You’ll then classify these objects based on their spectral, spatial, and contextual properties. This method is particularly effective for high-resolution imagery where individual pixels may not provide enough information for accurate classification.
OBIA offers several advantages over pixel-based methods.
You’ll find it’s better at handling the salt-and-pepper effect common in high-resolution images. It’s also more effective at identifying complex land cover types and can incorporate expert knowledge into the classification process. However, you’ll need to be aware that OBIA can be computationally intensive and requires careful parameter selection for ideal results.
Multitemporal Classification Approaches
While OBIA focuses on spatial relationships within a single image, multitemporal classification approaches expand the analysis across time.
You’ll find these techniques particularly useful when studying dynamic landscapes or seasonal changes. They involve analyzing a series of images taken at different times, allowing you to detect and classify land cover changes over periods ranging from days to years.
In multitemporal classification, you’ll typically use one of two main approaches: post-classification comparison or direct multi-date classification.
With post-classification comparison, you’ll classify each image separately and then compare the results to identify changes. This method’s advantage is that it minimizes atmospheric and sensor differences between dates.
In direct multi-date classification, you’ll analyze all images simultaneously, which can better capture subtle shifts and transformations.
You’ll need to ponder factors like image registration, atmospheric correction, and phenological cycles when applying these techniques.
Advanced methods include time series analysis and change vector analysis. These approaches can help you detect gradual changes, abrupt disturbances, or cyclical patterns in land cover.
Fusion of Multiple Data Sources
Data integration takes remote sensing to new heights. When you combine multiple data sources, you’re able to extract more thorough and accurate information about land cover.
This fusion approach allows you to overcome the limitations of individual sensors and leverage the strengths of different data types.
You’ll find that optical imagery, radar data, LiDAR, and hyperspectral information can be merged to create a richer representation of the Earth’s surface.
By integrating these diverse sources, you’ll capture both spectral and structural characteristics of land cover features. This multi-sensor approach enables you to distinguish between similar land cover types that might be indistinguishable using a single data source.
You can employ various fusion techniques, such as pixel-level, feature-level, or decision-level integration.
Each method offers unique advantages, depending on your specific classification goals.
When you’re working with multi-temporal data, you’ll also benefit from improved change detection capabilities.
By fusing data from different time periods, you’ll gain insights into land cover dynamics and temporal patterns.
This approach enhances your ability to monitor environmental changes, urban growth, and agricultural practices over time.
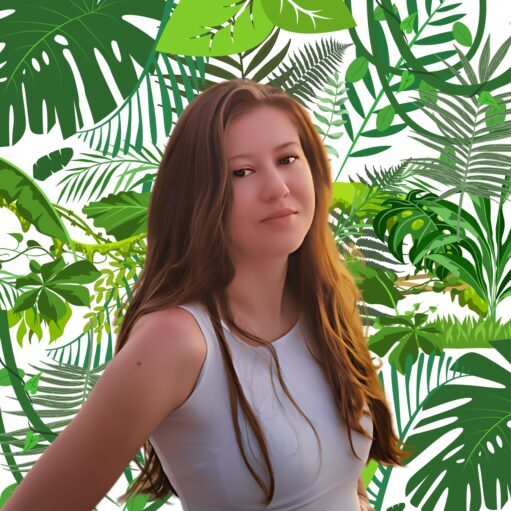
Erzsebet Frey (Eli Frey) is an ecologist and online entrepreneur with a Master of Science in Ecology from the University of Belgrade. Originally from Serbia, she has lived in Sri Lanka since 2017. Eli has worked internationally in countries like Oman, Brazil, Germany, and Sri Lanka. In 2018, she expanded into SEO and blogging, completing courses from UC Davis and Edinburgh. Eli has founded multiple websites focused on biology, ecology, environmental science, sustainable and simple living, and outdoor activities. She enjoys creating nature and simple living videos on YouTube and participates in speleology, diving, and hiking.
- WILDLIFE THEMED T-SHIRTS
Cute Hedgehog Embroidered: Love Wildlife, Protect Nature Wildlife conservation tees
$35.00